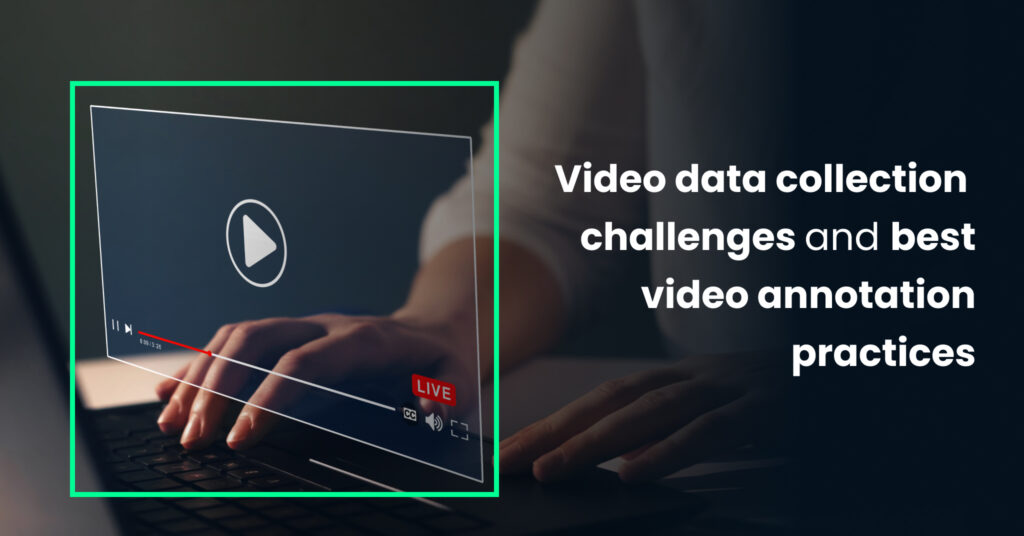
In recent years, the paradigm of video data has undergone a shift, transitioning from a mere conduit for moving images to a vital source of acquiring actionable insights. Although industries like surveillance, healthcare, automotive, entertainment, or retail have long leveraged video, its contemporary importance stems from its role as a primary data source for cutting-edge AI solutions.
Consider video data as the language of instruction for an AI system. Unlike humans who possess inherent knowledge, AI lacks innate understanding and requires thorough training. Video annotation functions as a guiding mentor, decoding the language of visuals and imparting essential knowledge to the AI.
However, this transformative journey from raw data to insights is not without its challenges. Collecting data, particularly in the video domain, introduces complexities that we will explore in this blog. We will also highlight the strategies to navigate the challenges along with the future trends in video annotation to ensure the seamless transformation of video data into a potent asset.
The complexity of gathering video data
1) Data volume
Video data consists of a continuous stream of frames, resulting in large datasets. Collecting and managing such vast volumes of data can strain storage resources and increase processing time, making efficient data collection challenging.
2) Diverse content
Videos encompass a wide range of content, from surveillance footage to medical procedures and sports events. Each type of content may require distinct annotation strategies and tools, necessitating a flexible and adaptable data collection approach.
3) Privacy concerns
Privacy considerations are paramount when collecting video data, especially in public spaces or involving individuals. Obtaining proper consent, ensuring data anonymization, and complying with privacy regulations can be complex and challenging.
4) Data synchronization
Videos often include multiple data streams, such as audio, depth, or infrared. Synchronizing these data streams accurately for annotation is crucial but can be technically challenging, particularly when dealing with complex sensor setups.
Strategies to overcome video data collection challenges for effective annotation
1) Preparing data for annotation
Data preprocessing before annotation is a crucial step in ensuring the accuracy and efficiency of the annotation process. Here’s why it matters:
- Data quality: Preprocessing helps improve data quality by addressing issues like noise, artifacts, or inconsistencies in the video data. Clean data is essential for accurate annotation.
- Alignment and synchronization: Properly aligning and synchronizing video data is vital, especially when dealing with multi-modal data sources or different camera angles. It ensures that annotations accurately correspond to the relevant frames or events.
- Noise reduction: Noise in video data can lead to false annotations. Cleaning involves filtering out irrelevant information or disturbances, such as motion blur or sensor noise, to improve annotation accuracy.
Example: In a self-driving car project, preprocessing may involve aligning video frames with GPS data to accurately annotate road signs and obstacles in real-time.
2) Choosing annotation types
Different annotation types serve distinct purposes in video analysis. Here are the key annotation types and their suitable applications:
- Object detection: It helps identify and locate objects within video frames. It’s ideal for tracking the position of objects like cars, pedestrians, or animals in surveillance or autonomous vehicle applications.
- Tracking: It focuses on following objects’ movements across frames. It’s useful for monitoring object trajectories, such as tracking a ball’s path in sports analysis.
- Segmentation: Here, annotations are assigned to each pixel in a frame to a specific object or region. This is valuable in medical imaging for segmenting organs in videos or in the film industry for special effects.
Example: In the medical context, segmentation might be employed to delineate tumors in MRI videos, while object detection could be used for counting people in a crowded event.
3) Annotation tools and software
Efficient annotation requires the right tools and software. Here’s an overview of popular video annotation tools and their functionalities:
- Labelbox: Labelbox is a versatile platform for annotating images and videos. It offers features like object tracking, keyframe selection, and collaboration tools for efficient video annotation.
- VGG Image Annotator (VIA): VIA is an open-source annotation tool with support for various annotation types, including object detection, segmentation, and keypoint annotation.
- OpenCV and Python Libraries: Libraries like OpenCV provide Python APIs for video annotation, making it possible to create custom annotation pipelines tailored to specific project needs.
4) Quality control and review
Quality control is paramount in video annotation to ensure the reliability of annotated data. Here’s why it’s significant and some tips for achieving it:
- Accuracy: Accurate annotations are essential for training machine learning models. Reviewing annotations and resolving discrepancies helps maintain accuracy.
- Consistency: Annotations should be consistent across frames or scenes. Establish annotation guidelines and conduct regular reviews to ensure consistency.
- Iterative review: Annotators can make mistakes or overlook details. Conducting iterative reviews and feedback sessions can help identify and rectify issues.
Example: In a video surveillance project, quality control may involve periodically reviewing object tracking annotations to ensure no objects are missed or incorrectly labeled.
Future trends
Image and video annotation are projected to play a pivotal role in the data labeling industry, with a remarkable CAGR of almost 28.9% expected from 2023 to 2030. Here are some emerging trends and technologies that are shaping the future of this field.
1) Multi-modal annotation
- Trend: Combining video data with other sensor data in the forms of audio, LiDAR, or GPS for comprehensive annotation.
- Impact: Enhanced context and richer insights for applications like autonomous vehicles, where multiple data sources are crucial for decision-making.
2) Privacy-preserving annotation
- Trend: Developing techniques for annotating sensitive or private video data without exposing personal information.
- Impact: Ensures compliance with data privacy regulations, making it feasible to use video data in healthcare, finance, and law enforcement without compromising privacy.
3) Semi-supervised learning
- Trend: Integration of semi-supervised learning techniques, where models learn from labeled and unlabeled data.
- Impact: Enables models to generalize better with less annotated data, which is beneficial for industries with limited access to annotated video data, such as agriculture.
4) Quality control and assurance
- Trend: The need for ensuring data accuracy and reliability before and during annotation will continue to rise.
- Impact: QA procedures involve rigorous checks, audits, and validation of annotations to guarantee their precision and consistency. This is crucial in healthcare, autonomous vehicles, and aerospace industries, where errors can have significant consequences.
5) Federated learning
- Trend: The adoption of federated learning allows multiple organizations to annotate collaboratively and train models without centralizing the data, addressing privacy and security concerns associated with sharing raw data.
- Impact: Accelerates advancements in fields like medical research and climate monitoring by enabling data collaboration while preserving data ownership.
These trends and technologies are poised to revolutionize how video data is collected and annotated, ultimately impacting various industries. By staying ahead of these developments, you can leverage video data more effectively, leading to improved decision-making, enhanced automation, and new possibilities across a wide range of applications.
Preparing for the future
As technology continues to advance and industries become more data-centric, staying at the forefront of video data utilization is crucial. Here are some strategies to prepare for the future:
A. Via internal changes
1) Continuous learning
Encourage a culture of continuous learning among your team members. Stay updated on the latest developments in video data collection, annotation techniques, and machine learning algorithms. Online courses, webinars, and conferences can be excellent resources.
2) Scalability
Prepare your infrastructure and data management systems to handle larger volumes of video data. Scalability will be essential as your data continues to grow.
3) Ethical considerations
Stay informed about evolving ethical and legal video data collection and privacy standards. Compliance with regulations will be crucial for the future.
4) Collaboration
Foster collaboration within your organization and with external partners. Cross-disciplinary teams and partnerships can lead to innovative solutions and insights.
5) Investment
Allocate resources for research and development. Investing in cutting-edge technology and talent will position your organization for future success.
6) Security
Enhance cybersecurity measures to protect valuable video data from potential threats. As data becomes more critical, security will be paramount.
B. Via external assistance
1) Partnering with data collection and annotation companies
Partnering with established data annotation service providers brings numerous benefits. These companies excel in both data collection and annotation, streamlining the entire workflow with efficient project management. With dedicated teams proficient in diverse annotation types, they offer expertise in every aspect. Whether scaling services, ensuring quality assurance, or complying with privacy regulations, these providers offer a holistic solution for enhanced project efficiency and quality.
2) Hiring freelancers
Another option is to hire freelance data annotators with specialized skills. Freelancers can offer flexibility and may be cost-effective for smaller projects. You can find freelancers on platforms like Upwork, Fiverr, Freelancer, or specialized data annotation marketplaces. When hiring freelancers, it’s essential to provide clear guidelines, establish communication channels for feedback, and conduct quality control to maintain the accuracy and consistency of annotations.