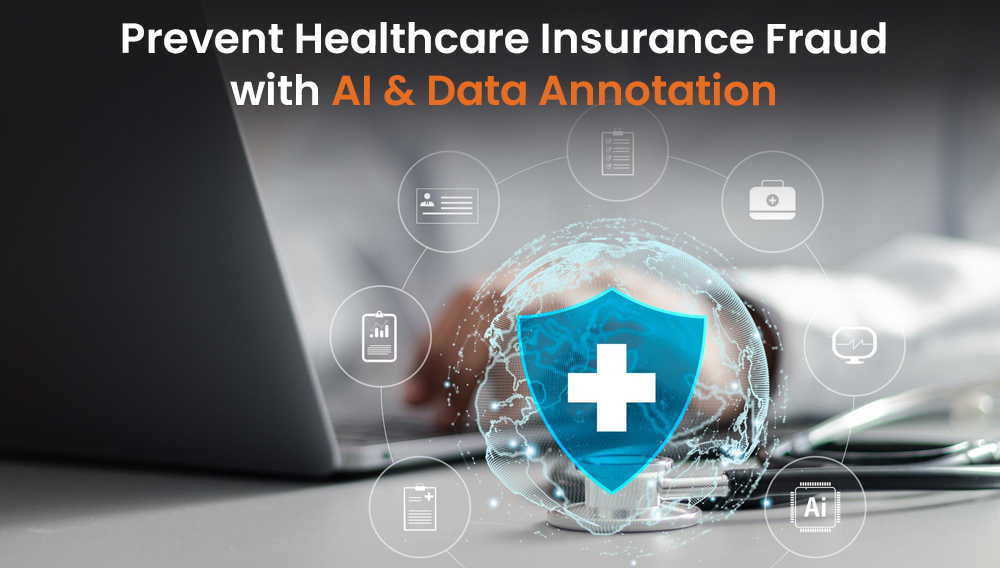
Insurance fraud is one of the most prevalent issues for healthcare providers, resulting in tens of billions in annual revenue losses, as reported by the National Health Care Anti-Fraud Association (NHCAA) in its last publicly available survey. The inefficiencies of manual claims processing only worsen the problem, highlighting the urgent need for innovative solutions. Fortunately, AI and machine learning models have revolutionized the industry, enabling healthcare insurers to detect and prevent fraud with remarkable efficiency and precision. Let’s explore the role of AI in the insurance industry and how it helps healthcare providers mitigate financial losses and manage claims effectively.
Table of content
The Role of AI in Healthcare Insurance Fraud Detection and Prevention
Identifying and preventing the payment of fraudulent claims as quickly and effectively as possible is a top priority for insurers to prevent significant revenue loss. Traditional fraud detection methods, which rely on predefined rules and human analysis, are time-consuming, hard to scale, and prone to false positives and fixed outcomes.
Hybrid intelligence (AI + human expertise) can revolutionize and speed up this process to improve operational efficiency and manage revenue cycles. Various aspects of the fraud detection process, such as compliance checks, data entry, verification, and claims categorization, can be automated, enabling subject matter experts to focus on more complex investigations. Additionally, by leveraging advanced machine learning algorithms, fraud analysts can identify sophisticated fraud schemes that may go unnoticed by traditional rule-based systems.
Common Use Cases of AI in the Insurance Industry
Predictive Modeling & Analytics:
Real-Time Fraud Detection:
Risk Scoring:
Network Analysis:
Leveraging artificial intelligence or machine learning models, insurers can analyze historical data, identify fraud patterns, and compare new claims against those known patterns to assess the risk and take preventive measures.
AI systems can monitor transactions and claims in real time, providing insurers with immediate alerts when suspicious activities are detected, allowing quicker responses to potential fraud.
AI/ML models can be used to assign risk scores to claims based on various factors, such as the provider’s history, the patient’s claim history, and the type of treatment. High-risk claims can be flagged for further investigation by an SIU (Special Investigation Unit).
AI systems can also map relationships between providers, patients, and claims to uncover fraudulent networks and collusion, such as provider-patient kickback schemes or illegal patient referral networks.
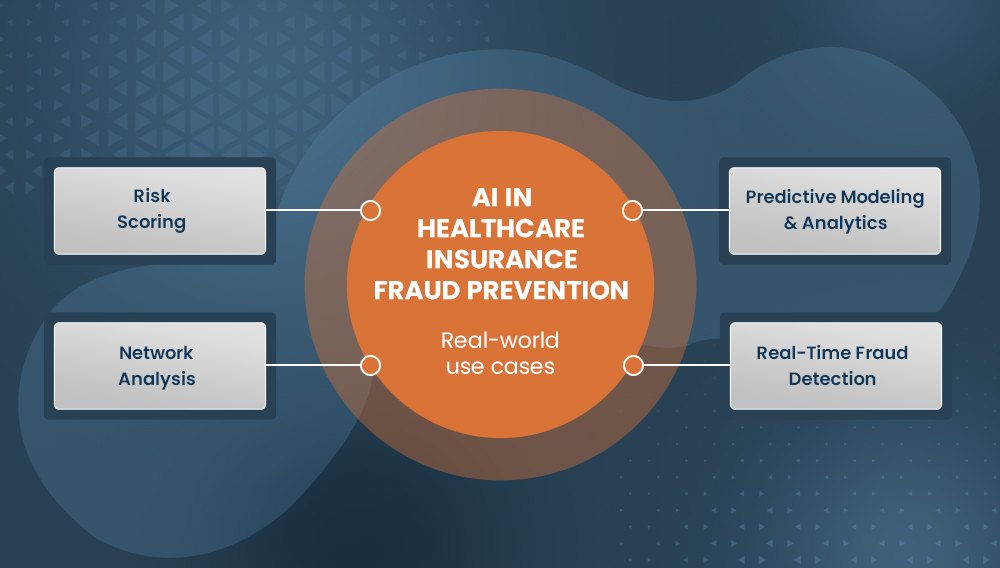
Types of Data Annotation Required for Creating Healthcare Fraud Detection Models
The accuracy of the healthcare insurance fraud detection systems depends primarily on its training data. To create effective fraud detection machine learning models that can identify various scenarios, it is essential to train them using diverse and precisely annotated data. Depending upon specific use cases and how the system works, different types of data labeling may be required.
- Text Classification and Sentiment Analysis
For fraud detection systems utilizing Optical Character Recognition capabilities, text classification and sentiment analysis annotation are critical. Leveraging NLP (Natural Language Processing), experienced annotators can precisely label key phrases in unstructured textual data, such as claim descriptions and medical reports, to identify fraud or related activities.
- Entity Recognition and Linking
Key entities in healthcare data, such as patient names, doctor names, dates, addresses, phone numbers, and medical procedures, can be labeled by skilled annotators. These labeled entities can be used to cross-reference and verify the authenticity of the claims and detect patterns that may indicate fraudulent activities.
- Image and Document Annotation
For cases involving procedures like medical imaging and radiology, X-rays, MRIs, and other necessary documents can be labeled. This helps verify whether the imaging results support the claimed procedures and confirm the authenticity of submitted documents, such as prescriptions and medical reports.
- Anomaly Detection
Unusual patterns or deviations from typical billing behavior in claims data can be detected and labeled as anomalies in the training data. It can help fraud detection models identify potential fraud, such as overbilling, upcoding, or billing for unnecessary procedures.
- Temporal Annotation
Timelines and sequences of medical events can be labeled to verify if the treatments and claims are chronologically plausible. This helps in identifying patterns that may indicate fraudulent activity, such as overlapping claims or implausible recovery times.
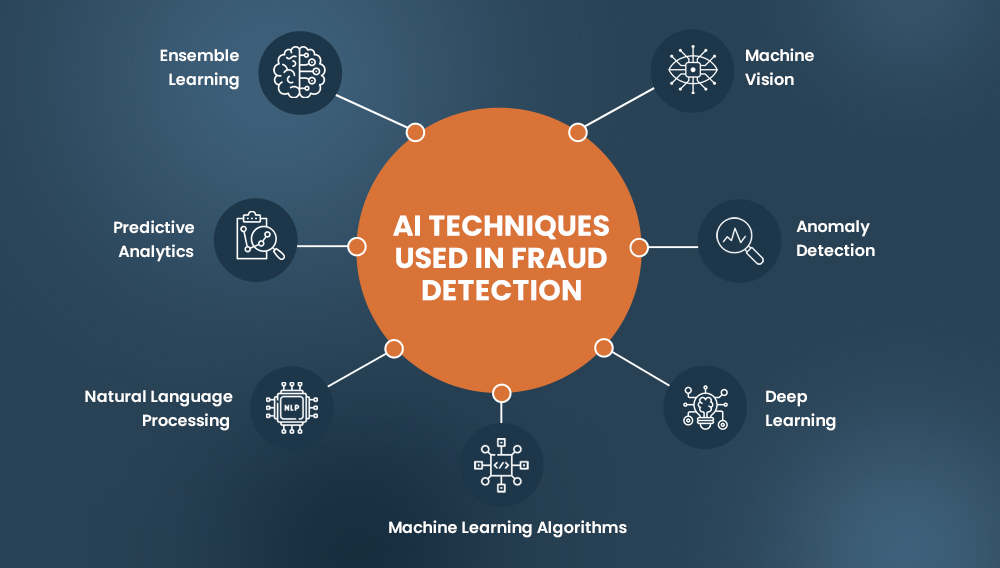
Challenges Involved in Implementing AI Fraud Detection Systems for Healthcare Insurance
While many recognized medical insurance providers and companies like Visanna and AllState are already harnessing the capabilities of AI and ML for fraud prevention and claim management, there are some noteworthy challenges associated with the implementation of these systems. It includes:
Data Quality and Availability Issues
Healthcare data is often fragmented, inconsistent, and incomplete because it is siloed across different providers, payers, and systems. Machine learning models rely heavily on high-quality, comprehensive datasets to identify fraudulent patterns. Poor data quality leads to unreliable models that may either miss fraudulent claims or incorrectly flag legitimate ones, undermining the effectiveness of the fraud detection system.
Explainability and Interpretability of AI/ML Models
AI and ML models, especially complex ones like deep learning, often operate as “black boxes,” making it difficult to understand their decision-making processes. In fraud detection, this lack of transparency is problematic. Insurers need to explain and justify decisions to stakeholders, including policyholders and regulatory bodies. Without clear insights into how these models work, gaining trust and ensuring compliance becomes challenging, potentially hindering the adoption of AI-driven fraud detection systems.
Regulatory and Privacy Concerns
It is crucial to ensure that healthcare fraud detection systems are designed to handle sensitive data responsibly, ensuring privacy is not compromised. Laws such as HIPAA in the United States impose strict requirements on how personal health information can be used and shared. Ensuring compliance with these regulations while effectively detecting fraud is both crucial and challenging.
Lack of Subject Matter Expertise
Insurance terminology and healthcare fraud schemes can be complex and constantly evolving, requiring deep domain knowledge. The shortage of professionals with combined expertise in healthcare, fraud analytics, and AI/ML can be a significant challenge for organizations. This expertise gap can delay the deployment of effective AI systems and result in suboptimal performance.
Role of Content Moderation in Creating Reliable Training Datasets for AI-Powered Fraud Detection Systems
To help AI systems better understand and prevent complex fraud scenarios, it is important to train the models on user-generated healthcare insurance fraud-related data. This can include user-submitted reports of suspicious transactions, comments or ratings suggesting potential fraud, discussions from public posts and forums, posts on social media where users describe their experience with insurance fraud, etc.
Content moderation experts can collect and review such occurrences to highlight complex nuances. The curated data can then be used to train AI models to learn from real-world patterns. Once the AI systems are able to identify anomalies in user behavior patterns, they can help with quick identification and prevention of insurance fraud.
Data Annotation Outsourcing: A Strategic Approach to Acquire Training Datasets for Medical Fraud Detection Systems
It is crucial to recognize that for healthcare insurance fraud prevention, AI models are only effective when trained on high-quality annotated data. For businesses lacking the resources or expertise to acquire such datasets, outsourcing data annotation services becomes a practical solution.
By partnering with reputed data labeling service provider, healthcare insurance firms can ensure:
- Scalability and Efficiency: With a scalable workforce and agile workflow, outsourcing companies support large-scale annotation projects, ensuring the timely delivery of labeled datasets.
- Accuracy and Consistency: Content moderation outsourcing companies typically hold essential certifications, such as ISO, to ensure data accuracy and security. They employ a rigorous QA process that includes multiple rounds of review, statistical sampling, and inter-annotator agreement checks.
- Subject Matter Expertise: Well-versed in healthcare insurance terminology, coding practices, and regulatory compliance, experts ensure that the training data is accurately labeled and annotated, capturing the nuances and complexities of medical fraud scenarios.
- Cost-Effectiveness: With flexible engagement models, outsourcing companies help businesses reduce overhead costs associated with maintaining in-house annotation teams.
Struggling to manage your data labeling tasks in-house?
Let our subject matter experts handle them with precision and efficiency.
On a Concluding Note
While intelligent fraud prevention systems are the need for the hour, their accuracy and effectiveness depend on high-quality training datasets. By using smart technology and carefully labeled data, healthcare insurance firms can catch fraudulent activities early on, protecting both themselves and their customers from financial loss.